Privacy by Design for Image Processing
Learn how to embrace privacy by design into your image capture and processing workflow to proactively comply with the GDPR and avoid fines and other risks.
23 July 2020, by Mario Sabatino RiontinoAsk a question
We are living in an era dominated by digital innovation. Data has proved to have immense potential, therefore itâs captured, stored, and shared at an unimaginable pace and size.
However, weâve recently witnessed a lot of media revelations on data scandals, breaches, suits etc., involving some of the worldâs biggest corporations. As a result, protecting privacy resulted more and more in the spotlight, affecting the citizensâ sentiment about data. For example, according to a survey conducted in 2016 by Deloitte, 81% of the respondents from the US had pointed out that they feel they have lost control over the way their personal data is being collected and used.
Consequently, the demand for privacy has grown at a rapid pace. Traditional approaches - which consider privacy as a separate implementation or a secondary feature - have proved to be unfeasible for companies and organizations due to the increasing costs and volume of data to deal with.
What is Privacy by Design?
Implement technical and organisational measures at the earliest stages of the product/service development.
Privacy by Design is among one of the most innovative approaches in data protection. It recommends that companies should implement technical and organisational measures at the earliest stages of the product/service development, to ensure privacy right from the start.
According to the framework developed by Dr. Ann Cavoukian, it is based on 7 fundamental principles:
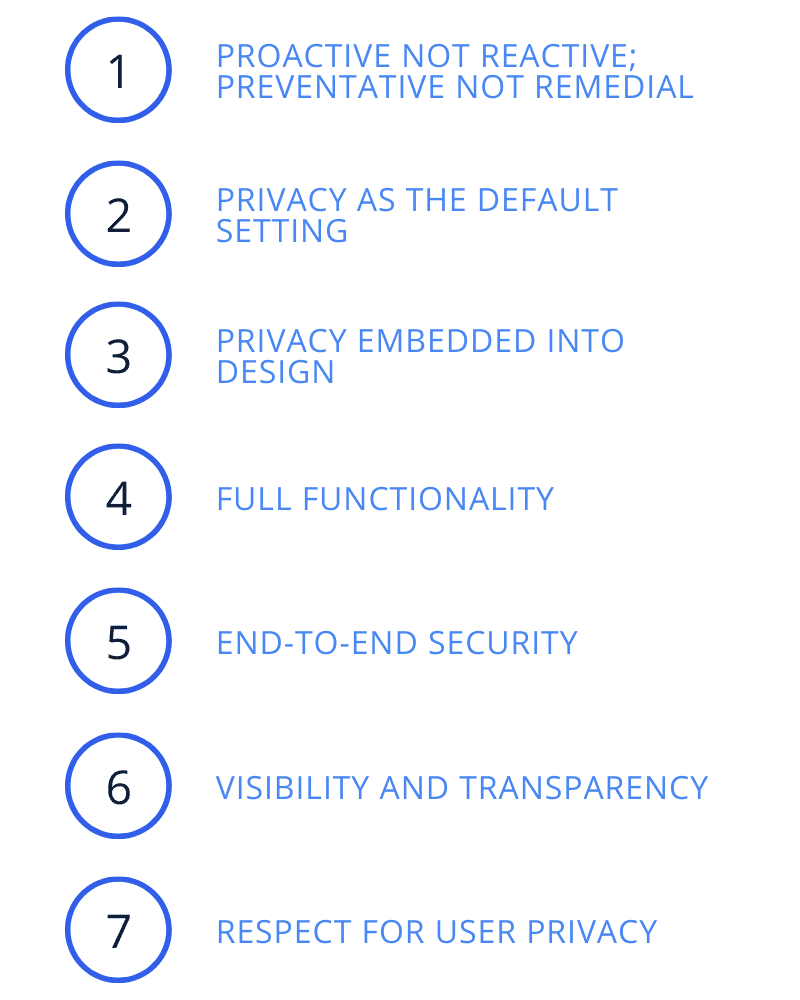
- Proactive not reactive: Anticipate, identify, prevent and take action before the data breach, not afterward.
- Privacy as the default setting: Ensure that personal data is automatically protected in all IT systems or business practices.
- Privacy embedded into design: Privacy measures should not be add-ons, but fully-integrated part of the system.
- Full functionality: Privacy by Design employs a âwin-winâ approach, since both privacy and product functionalities are preserved.
- End-to-end security: All data should be securely retained as needed and destroyed when no longer needed.
- Visibility and transparency: Be transparent on business practices and technologies that are operating according to the objectives, and subject to independent verification.
- Respect for user privacy: Individual privacy must be user-friendly and supported by strong privacy standards.
Image and video data
Image and video data are no exception in regards to containing personal information. Faces and bodies are among the most fundamental and highly visible elements of our identity. Hence, they fall under the definition of personal data according to the art. 4 of the GDPR and need to be protected accordingly.
Applying Privacy by Design approaches should be considered by any industry that collects images and videos using cameras, CCTV, drones and sensors. Some of the use-cases we could list are:
- Self-Driving Vehicles
- Drones
- Mobile Mapping
- Video surveillance
- Computer vision
- ... and many others
Use-case #1: Mobile Mapping
In the specific case of Mobile Mapping, Privacy by Design principle can be embraced from the planning (data categories and how to collect them) to the processing phase (e.g. by using anonymization techniques).
Planning plays a fundamental role. When it comes to data collection, GDPR suggests to follow the so-called data minimization principle, i.e. limiting data collection to what is necessary while ensuring full functionality.
Nevertheless, using data minimization is not enough to comply with GDPR. During the mapping phase, Mobile Mapping Systems (MMS) collects data such as location information, point clouds, as well as street-level and aerial imagery. Thus faces, bodies, license plates and coordinates might be captured, requiring explicit consent or anonymization.
An additional implication comes from data storage, i.e. choosing between cloud vs. on-premise solutions. GDPR-certified cloud providers might offer end-to-end security by default, while hosting your own data center requires additional effort to achieve similar standards.
If you are conducting mobile mapping, or selling or renting mobile mapping equipment, you can integrate Celanturâs anonymization solution to offer state-of-art privacy standards.
Use-case #2: Self-Driving Vehicles
A study from IBM showed that finding a software error in the design phase costs less than a sixth of the cost of finding it in the implementation phase, 15 times less than during the testing phase and 1/100th of the cost as in product maintenance.
Oftentimes, privacy is designed separately from the rest of the automobile or left as an integration task during manufacturing, instead of being addressed as early as possible.
Autonomous driving involves complex algorithms, trained using driversâ information, location tracking and sensor data. Of all this data, a great quantity will be considered personal data, so that the General Data Protection Regulation (GDPR) will then apply to autonomous vehicles.
Privacy by Design could be embraced from idea definition (requirements, risk assessment, etc.) to the validation phase (field test and proof of safety).
Despite comprehensive simulation models across the entire design lifecycle are blooming, real-world data still matter. Data collection is essential to generating possible scenarios of everything that can happen on the road.
As for the previous use-case, not all data collected is actually necessary from a technical perspective to enable autonomous driving. This applies, for example, to license plate numbers for the purposes of recognising obstacles and yet, data minimisation comes again into consideration to reduce the amount of gathered data.
At the same time, the processing of all these data is not expected to be carried out by a single data controller who has to comply with the data protection requirements, but possibly shared with third-parties. Hence, data requires to be anonymized before being shared.
Final Questions to Consider
- What steps are you taking to address the growing requirements for privacy?
- How are you managing access, ownership, and protection of the data that is collected?
- How is your organization working across the entire workflow, in a way that privacy is implemented as early as possible?
About Celantur
Technology
Celantur offers fully-automated image and video anonymization solutions to help companies and organizations comply with privacy laws. Our technology detects several objects to be anonymized such as faces, bodies, license plates, vehicles and facades, and automatically blurs them:
â We anonymize all kinds of RGB-imagery: planar, panorama and video
â Our cloud platform is capable of anonymizing around 200.000 panoramas per day and 90.000 video frames per hour.
â Industry-grade anonymization quality: detection rate up to 99%
Data Protection Standards
Data protection is our core business. That's why, to operate as a data processor, we have robust measures in place to comply with the GDPR and other data protection laws:
- Images are processed in GDPR-certified data centres in the European Union
- External Data Protection Officer at service
- All data and storage devices are encrypted
- Annual Data Protection Audit
- Up-to-date Documentation: Technical and Organizational Measures ("TOMs"), Records of Processing Activities and Data Processing Agreement
Ask us Anything. We'll get back to you shortly